Stable Diffusionの仕組みについてご存知ですか?
プロンプトを入れると画像が生成される…その内部を説明できるとかっこいいですよね。
こちらの講演で使用したスライドを白井CEOから頂いたので一部公開いたします。
https://note.com/aicu/n/n8d4575bcf026
画像生成AIの誕生と変遷(2)画像生成技術の歴史年表
https://note.com/o_ob/n/n971483495ef3
画像生成AIの誕生と変遷(4) 画像生成AIの2014–2024におきたパラダイムシフト
https://note.com/o_ob/n/n3c1d8523cf68
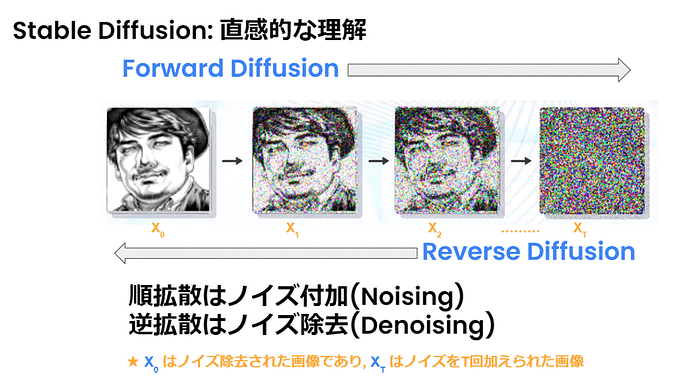
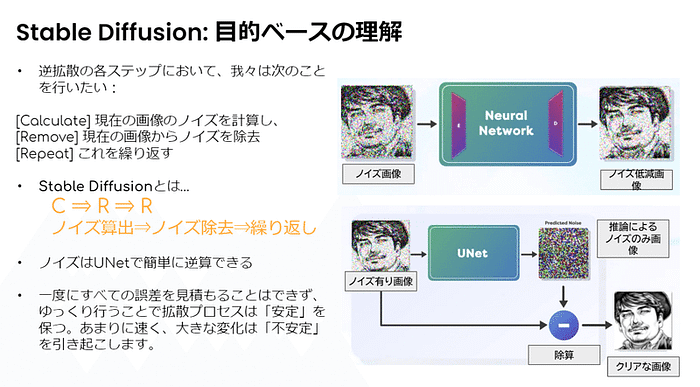
Stable Diffusionの仕組み(入門編)
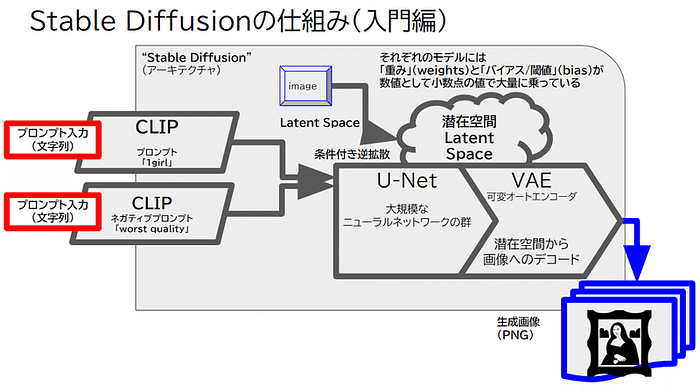
CLIPとは画像とテキストの関係だけを学んでいます。
CLIP (Contrastive Language-Image Pretraining), Predict the most relevant text snippet given an image
https://github.com/openai/CLIP
実はネガティブプロンプトはStable Diffusionが公開されてからAUTOMATIC111で生まれました。
https://github.com/AUTOMATIC1111/stable-diffusion-webui/wiki/Negative-prompt
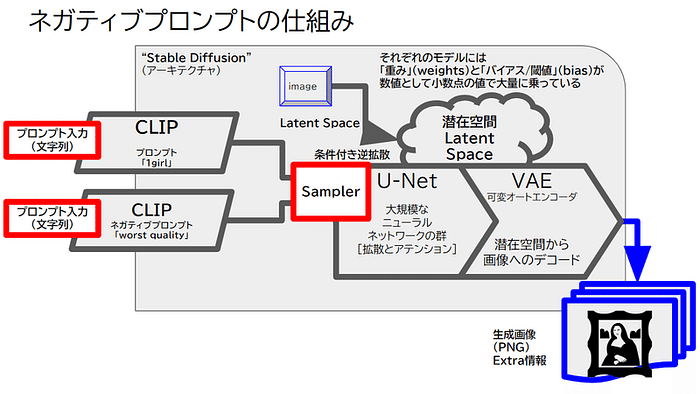
ネガティブプロンプトは、正プロンプトと同じ仕組みでCLIPを持ち、サンプラーが条件付き逆拡散をする際にUNETの左側に入ります。
画像のLatent Space化はちょうどZIP圧縮のような超圧縮で、フロート列が並んでいるweight&biasです。VAEを使うことで画像に展開できます。
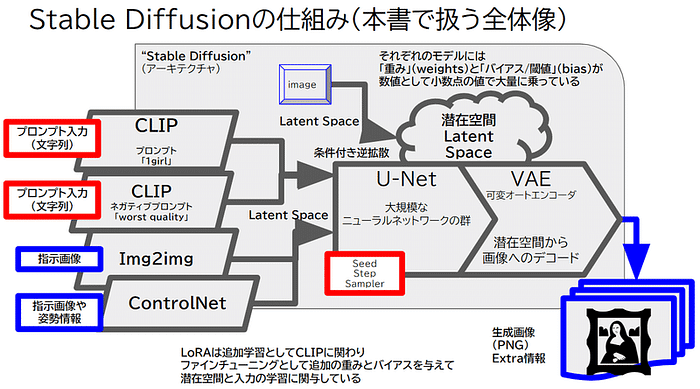
ImageToImageやControlNetも、基本的にはLatent SpaceでUNETの左側に入ります。
UNetについて補足
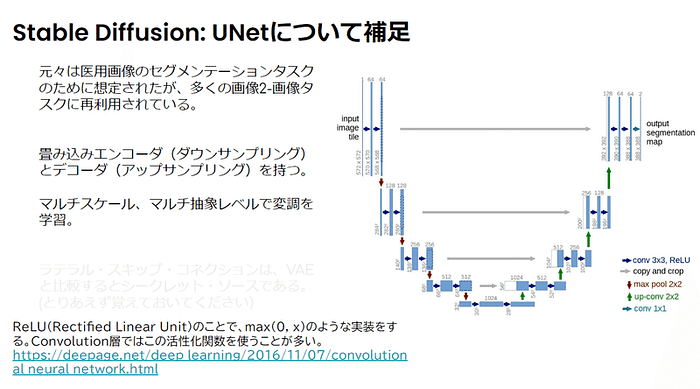
UNETは元々は医用画像のセグメンテーションタスクのために想定されたが、多くの画像2-画像タスクに再利用されている。畳み込みエンコーダ(ダウンサンプリング)とデコーダ(アップサンプリング)を持つ。
マルチスケール、マルチ抽象レベルで変調を学習。
ReLU(Rectified Linear Unit)のことで、max(0, x)のような実装をする。Convolution層ではこの活性化関数を使うことが多い。
https://deepage.net/deep_learning/2016/11/07/convolutional_neural_network.html
https://lmb.informatik.uni-freiburg.de/people/ronneber/u-net/
画像生成AI Stable Diffusion スタートガイドより。
Originally published at https://note.com on May 30, 2024.
Comments